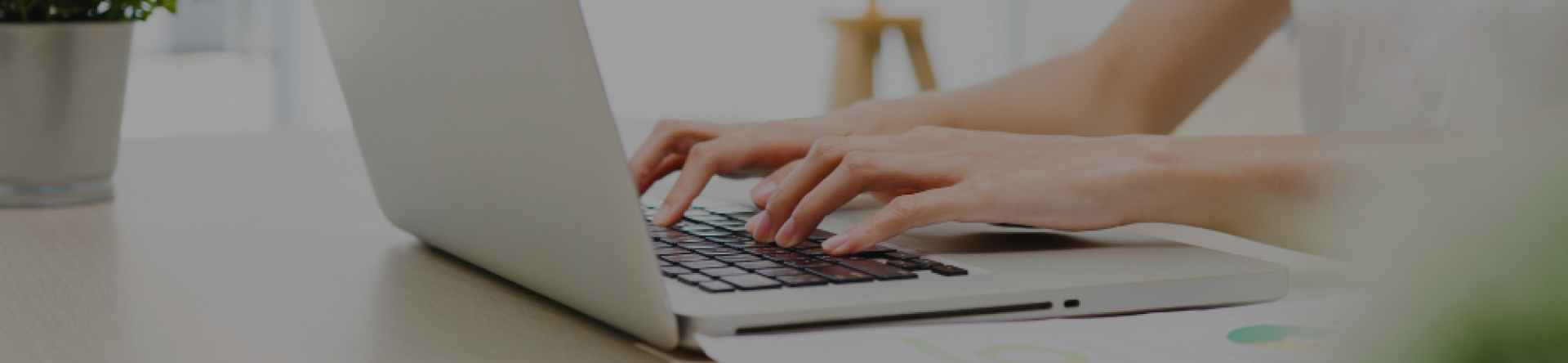
HOME > Blog
Blog
Blog Image
The Metaverse is coming! What does it mean for your business?
Rapid digitization of brands has led to major technological leaps...
Blog Image
Another Blog Post Title Here
Sample description for another blog post...
Blog Image
Third Blog Post Example
Description for the third blog post example...